This is a fixed-text formatted version of a Jupyter notebook
- Try online
- You can contribute with your own notebooks in this GitHub repository.
- Source files: spectrum_simulation.ipynb | spectrum_simulation.py
Spectrum simulation with Gammapy¶
Introduction¶
This notebook explains how to use the functions and classes in gammapy.spectrum in order to simulate and fit spectra.
First, we will simulate and fit a pure power law without any background. Than we will add a power law shaped background component. Finally, we will see how to simulate and fit a user defined model. For all scenarios a toy detector will be simulated. For an example using real CTA IRFs, checkout this notebook.
The following clases will be used:
Setup¶
Same procedure as in every script …
[1]:
%matplotlib inline
import matplotlib.pyplot as plt
[2]:
import numpy as np
import astropy.units as u
from gammapy.irf import EnergyDispersion, EffectiveAreaTable
from gammapy.spectrum import SpectrumSimulation, SpectrumFit
from gammapy.spectrum.models import PowerLaw
Create detector¶
For the sake of self consistency of this tutorial, we will simulate a simple detector. For a real application you would want to replace this part of the code with loading the IRFs or your detector.
[3]:
e_true = np.logspace(-2, 2.5, 109) * u.TeV
e_reco = np.logspace(-2, 2, 79) * u.TeV
edisp = EnergyDispersion.from_gauss(
e_true=e_true, e_reco=e_reco, sigma=0.2, bias=0
)
aeff = EffectiveAreaTable.from_parametrization(energy=e_true)
fig, axes = plt.subplots(1, 2, figsize=(12, 6))
edisp.plot_matrix(ax=axes[0])
aeff.plot(ax=axes[1]);
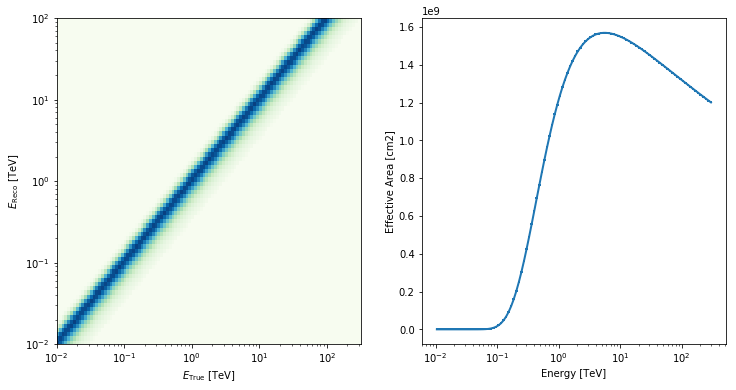
Power law¶
In this section we will simulate one observation using a power law model.
[4]:
pwl = PowerLaw(
index=2.3, amplitude=1e-11 * u.Unit("cm-2 s-1 TeV-1"), reference=1 * u.TeV
)
print(pwl)
PowerLaw
Parameters:
name value error unit min max frozen
--------- --------- ----- -------------- --- --- ------
index 2.300e+00 nan nan nan False
amplitude 1.000e-11 nan cm-2 s-1 TeV-1 nan nan False
reference 1.000e+00 nan TeV nan nan True
[5]:
livetime = 2 * u.h
sim = SpectrumSimulation(
aeff=aeff, edisp=edisp, source_model=pwl, livetime=livetime
)
sim.simulate_obs(seed=2309, obs_id=1)
print(sim.obs)
*** Observation summary report ***
Observation Id: 1
Livetime: 2.000 h
On events: 339
Off events: 0
Alpha: 1.000
Bkg events in On region: 0.00
Excess: 339.00
Gamma rate: 2.83 1 / min
Bkg rate: 0.00 1 / min
Sigma: nan
energy range: 0.01 TeV - 100.00 TeV
[6]:
fit = SpectrumFit(obs_list=sim.obs, model=pwl.copy(), stat="cash")
fit.fit_range = [1, 10] * u.TeV
fit.run()
print(fit.result[0])
Fit result info
---------------
Model: PowerLaw
Parameters:
name value error unit min max frozen
--------- --------- --------- -------------- --- --- ------
index 2.259e+00 2.191e-01 nan nan False
amplitude 9.255e-12 1.754e-12 cm-2 s-1 TeV-1 nan nan False
reference 1.000e+00 0.000e+00 TeV nan nan True
Covariance:
name index amplitude reference
--------- --------- --------- ---------
index 4.802e-02 2.984e-13 0.000e+00
amplitude 2.984e-13 3.077e-24 0.000e+00
reference 0.000e+00 0.000e+00 0.000e+00
Statistic: -74.059 (cash)
Fit Range: [1. 9.42668455] TeV
Include background¶
In this section we will include a background component. Furthermore, we will also simulate more than one observation and fit each one individuallt in order to get average fit results.
[7]:
bkg_model = PowerLaw(
index=2.5, amplitude=1e-11 * u.Unit("cm-2 s-1 TeV-1"), reference=1 * u.TeV
)
[8]:
%%time
n_obs = 30
seeds = np.arange(n_obs)
sim = SpectrumSimulation(
aeff=aeff,
edisp=edisp,
source_model=pwl,
livetime=livetime,
background_model=bkg_model,
alpha=0.2,
)
sim.run(seeds)
print(sim.result)
print(sim.result[0])
SpectrumObservationList
Number of observations: 30
*** Observation summary report ***
Observation Id: 0
Livetime: 2.000 h
On events: 733
Off events: 1915
Alpha: 0.200
Bkg events in On region: 383.00
Excess: 350.00
Excess / Background: 0.91
Gamma rate: 2.92 1 / min
Bkg rate: 0.04 1 / min
Sigma: 14.17
energy range: 0.01 TeV - 100.00 TeV
CPU times: user 298 ms, sys: 4.3 ms, total: 302 ms
Wall time: 151 ms
Before moving on to the fit let’s have a look at the simulated observations
[9]:
n_on = [obs.total_stats.n_on for obs in sim.result]
n_off = [obs.total_stats.n_off for obs in sim.result]
excess = [obs.total_stats.excess for obs in sim.result]
fix, axes = plt.subplots(1, 3, figsize=(12, 4))
axes[0].hist(n_on)
axes[0].set_xlabel("n_on")
axes[1].hist(n_off)
axes[1].set_xlabel("n_off")
axes[2].hist(excess)
axes[2].set_xlabel("excess");
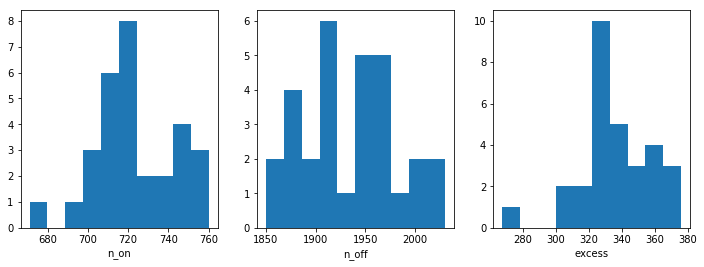
[10]:
%%time
results = []
for obs in sim.result:
fit = SpectrumFit(obs, pwl.copy(), stat="wstat")
fit.optimize()
results.append(
{
"index": fit.result[0].model.parameters["index"].value,
"amplitude": fit.result[0].model.parameters["amplitude"].value,
}
)
CPU times: user 2.67 s, sys: 9.33 ms, total: 2.68 s
Wall time: 1.34 s
[11]:
index = np.array([_["index"] for _ in results])
plt.hist(index, bins=10)
print("spectral index: {:.2f} +/- {:.2f}".format(index.mean(), index.std()))
spectral index: 2.31 +/- 0.09
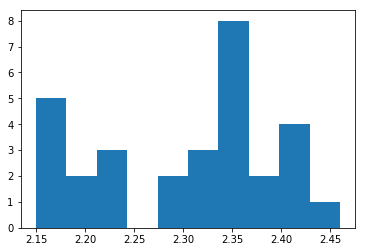
Exercises¶
- Fit a pure power law and the user define model to the observation you just simulated. You can start with the user defined model described in the spectrum_models.ipynb notebook.
- Vary the observation lifetime and see when you can distinguish the two models (Hint: You get the final likelihood of a fit from
fit.result[0].statval
).
What’s next¶
In this tutorial we learnd how to simulate and fit data using a toy detector. Go to gammapy.spectrum to see what else you can do with gammapy.